Trademarks & Copyrights
The Challenges of Using Protected Material in AI Training
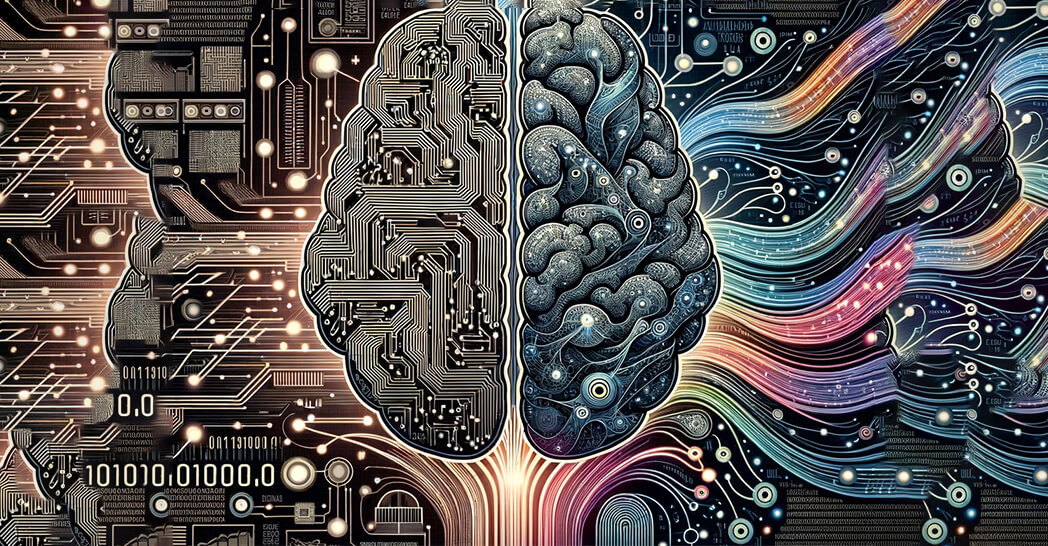
In the rapidly evolving landscape of artificial intelligence (AI), data serves as the lifeblood that fuels machine learning models. However, the use of copyrighted, trademarked, or otherwise protected material for training these models has opened a Pandora’s box of legal complexities. A recent class action lawsuit brought against OpenAI by the Author’s Guild and a class of individual plaintiffs, including renowned author George R.R. Martin, underscores the urgency of this issue. The lawsuit alleges that OpenAI “copied Plaintiffs’ works wholesale, without permission or consideration” and fed them into their large language models (LLMs).
These LLMs, according to the complaint, not only “endanger fiction writers’ ability to make a living” but also “can spit out derivative works” that threaten to erode the market for the original works. The lawsuit further accuses OpenAI of “systematic theft on a mass scale” and seeks damages for “the lost opportunity to license their works.” As these technologies become integral to commercial enterprises, lawsuits like this one will serve as a bellwether, urging us to examine the legal and ethical ramifications of using protected material in AI training. The central question that the business community will need to answer is what the legal implications of using copyrighted, trademarked, or otherwise protected material to train an AI model will be.
The Importance of Data in AI
In the realm of artificial intelligence, data is not merely an ancillary component; it is the very cornerstone upon which machine learning models are built and refined. The development of an AI application is a multi-stage process that begins with the acquisition of a large amount of data. This data is then segmented into various sets for training, testing, and evaluation. Throughout this intricate process, data and data labeling companies play a pivotal role, ensuring that the AI models are trained on accurate and relevant data.
Creating appropriate datasets and data pipelines for model training is emerging as the most formidable challenge in AI development. Data labeling companies facilitate the access and utilization of data, ensuring that the datasets are accurate, current, and consistent. This is crucial for the reliability and accuracy of AI models and applications. When data is appropriately labeled, it gains additional context through the use of semantic algorithms, becoming more useful for AI development. As a result, data-as-a-service firms are emerging as a reliable and compliance friendly option for AI developers in need of accurate and legally ironclad datasets.
While data is indispensable for AI development, it is imperative that it be collected, stored, and maintained securely to protect privacy and comply with legal and ethical requirements. The recent class action lawsuit against OpenAI demonstrates how the legal community is still grappling with the fundamental question of how the ethical provenance of data should take place, while the tech firms that are at the forefront of the AI revolution move deeper into the development process with reckless abandon. This model of innovation is the norm in the tech community, where the consequences of major disruptions (even the positive ones) often lag behind the cycle of invention and adoption.
The Legal Landscape
As these technologies reach wider and wider adoption, the legal frameworks governing them are coming under deep scrutiny. Copyright law, trademark law, and patent law are fields full of tests and standards that are based on precedent and smell tests. AI products complicate the pre-existing legal landscape, because they challenge the core ideas that make these tests viable in litigation. Many of the established evaluation methods used in the courts today are from the pre-digital era, and some would say the courts have yet to adequately catch up with the tech industry’s relationship with intellectual property more broadly. These frameworks often employ heuristic evaluations, such as the “transformative use” test in copyright law or the “likelihood of confusion” test in trademark law, to determine the legality of a particular action. However, the advent of AI technologies has thrown a proverbial wrench into these well-oiled legal machines.
AI products, particularly machine learning models, challenge the very core ideas that make these legal tests viable in litigation. For instance, the “transformative use” test in copyright law assesses whether a new work adds something new or alters the original work in a way that contributes a new expression, meaning, or message. But how do we apply this test to an AI model that can generate text, images, or even music based on copyrighted material? Is the AI’s output transformative, or is it merely a derivative work that infringes upon the original creator’s rights? Similarly, the “likelihood of confusion” test in trademark law becomes nebulous when an AI model generates content that could potentially mimic or dilute a trademarked brand or logo.
Some jurisdictions abroad, such as the United Kingdom and the European Union have already begun to carve out exceptions to their intellectual property protections frameworks. These emergent trends abroad may serve as the guinea pig for the United States’ eventual reconciliation of AI and IP law.
Case Study: Author’s Guild v. Google
In 2019, the Author’s Guild took Google to court, alleging that the company’s book-scanning project constituted a massive copyright infringement. Google defended itself by invoking the “fair use” doctrine, arguing that its actions were transformative and provided a public benefit by making books searchable. The court ultimately sided with Google, stating that the project provided a “new and transformative use” of the copyrighted material and did not offer a market substitute for the original works.
This case sets a significant precedent that could be consequential for the class action lawsuit against OpenAI. If OpenAI can successfully argue that its use of copyrighted material is transformative and serves a broader public interest—much like Google did—it may find legal grounds to defend its actions.
Fair Use Doctrine: A Possible Safe Harbor?
The fair use doctrine is generally assessed based on four factors: the purpose and character of the use, the nature of the copyrighted work, the amount and substantiality of the portion used, and the effect of the use on the market value of the original work. In Author’s Guild v. Google, the court found that Google’s use was “transformative,” as it provided a new and beneficial function—namely, making books searchable—that did not substitute for the original works. This transformative aspect weighed heavily in Google’s favor and could serve as a crucial point of consideration in future AI-related cases.
If an AI or machine learning model can demonstrate that its use of copyrighted material serves a transformative purpose, that could weigh in its favor. However, the definition of “transformative” in the context of AI remains an open question. Is it transformative for an AI model to learn human language by ingesting copyrighted books, or is this simply a new form of reproduction?
Courts will likely scrutinize whether the amount of copyrighted material used is reasonable in relation to the transformative purpose served. This is likely to create serious challenges for LLMs and other AI products that use very large quantities of copyrighted content to fuel their products’ offerings. Machine learning models like OpenAI’s GPT-4 are trained on enormous datasets that include significant proportions of copyrighted works compared to their overall datasets. Unlike Google’s book-scanning project, which used snippets of books to create a searchable index, machine learning models ingest and process the full text of works to understand context, semantics, and other linguistic nuances. The sheer volume of copyrighted material used could potentially weigh against OpenAI in a legal assessment based on the “amount and substantiality” factor.
One of the most contentious issues in fair use doctrine is the effect of the offending media’s use of copyrighted material on the market value of the original works. If an AI model generates outputs that could serve as market substitutes for the original, copyrighted works, this could weigh against a finding of fair use. Courts may also consider whether the copyrighted material is more factual or creative, with the use of factual works more likely to be considered fair use. However, AI’s ability to generate creative outputs based on factual data complicates this factor.
Possible Consequences for IP Infringement
It is crucial to also consider the potential consequences of intellectual property (IP) infringement. These consequences are not merely punitive but can have far-reaching implications for AI developers. Knowing the risks before they are imminent is an important step towards the creation of a compliance-first company culture, which saves time, energy, and money throughout the life of your business.
The most immediate consequence of IP infringement is legal action, as exemplified by the class action lawsuit against OpenAI. Companies found guilty of infringement may be subject to hefty fines, damages, and even injunctive relief, which could require the cessation of certain business activities. The financial ramifications can be severe, potentially running into millions of dollars, depending on the scale of infringement and the market value of the copyrighted material.
While fines and damages can be substantial, there are other long-term legal consequences that can be even more debilitating for developers. Injunctions, for instance, could halt the use of certain algorithms or even require the complete shutdown of specific services, disrupting business operations and leading to a loss of competitive advantage. Moreover, a legal defeat in one jurisdiction could set a precedent for similar lawsuits in other jurisdictions, triggering a cascade of challenges and associated costs in a legal environment where the odds are stacked against the offending developer. The court may also require the offender to undergo rigorous legal audits and compliance checks, adding another layer of operational complexity. These legal ramifications can divert significant resources and focus away from innovation and growth, affecting the company’s market position and long-term viability.
Beyond the financial penalties, IP infringement can lead to a significant loss of reputation for AI developers. In an industry where trust is paramount, especially given the transformative and pervasive nature of AI technologies, a loss of reputation can have long-lasting effects. A loss of reputation can affect investor confidence, leading to a decline in the firm’s valuation and making it challenging to secure future investments. Customer relationships, too, can suffer. In an age where consumers are increasingly concerned about ethical consumption, a reputation tarnished by high-profile IP infringement can lead to loss of customer loyalty and market share.
Ironically, IP infringement can also stifle innovation—the very thing that AI aims to foster. Legal uncertainties and the threat of litigation can make AI developers overly cautious, hindering them from pushing the boundaries of what is technologically possible. This could slow down the pace of AI advancements, affecting various sectors, from healthcare and education to transportation and entertainment.
Best Practices for AI Developers
As AI technologies continue to advance, developers must find the right balance of innovation and caution at the heart of a complex and rapidly evolving legal debate. The case against OpenAI serves as a cautionary tale, highlighting the need for due diligence and responsible practices. Here are some best practices for AI developers to consider:
Be aware of the legal landscape.
AI is a rapidly evolving field, and the law is struggling to keep up. As a result, there are a lot of legal gray areas when it comes to AI. It’s important to be aware of the laws that may apply to your work, and to consult with an attorney if you have any questions.
Document your work.
It’s important to carefully manage documentation and technical information as you develop AI systems. Not only will this process help you to track your progress, it will also provide you with a record of your decisions that shows the level of modification intrinsic to the development process. This documentation can be helpful if you are ever challenged about your work, and you plan to draw on the fair use doctrine.
Collect data responsibly.
Since AI systems are limited by the quality and quantity of the data used to train them, the pedigree of your training set is critically important. With that in mind, it is very important that the necessary training data is obtained while observing the intellectual property rights of its original owners, to avoid major compliance implications down the road. Wherever possible, obtain data directly from a trusted source and make sure that source gives consent for your intended usage of the dataset. For certain projects, consider using open-source datasets that are not subject to intellectual property restrictions.
Conclusion
Artificial intelligence is poised to redefine the global economy, but the intrinsic legal and ethical challenges stemming from this revolutionary field’s widespread use of copyrighted, trademarked, or otherwise protected material in its training data may derail that progress before it is fully realized. Ongoing litigation against OpenAI may yield critical insight into the court system’s plan to navigate the complexities of intellectual property and artificial intelligence, but the jury’s still out. Responsible developers can prudently avoid getting stuck in this legal quagmire by avoiding overreliance on protected material, or by obtaining robust consent to use any data that is necessary to bring an AI offering to market.